Bài giảng Đa phương tiện và các ứng dụng giải trí - Chương III: Ảnh - Lê Tấn Hùng
Người học sẽ:
Được trang bị kiến thức về quá trình tạo ảnh,
biểu diễn, nén và lưu trữ ảnh
Được giới thiệu một số kỹ thuật xử lý ảnh cơ
bản, một số công cụ xử lý
Sau khi kết thúc chương, người học :
Nắm được kiến thức cơ bản của tạo ảnh, biểu
diễn, lưu trữ ảnh
Biết vận dụng một số kỹ thuật, công cụ xử lý
ảnh để thực hành xử lý một số ảnh cụ thể
Bạn đang xem 20 trang mẫu của tài liệu "Bài giảng Đa phương tiện và các ứng dụng giải trí - Chương III: Ảnh - Lê Tấn Hùng", để tải tài liệu gốc về máy hãy click vào nút Download ở trên
Tóm tắt nội dung tài liệu: Bài giảng Đa phương tiện và các ứng dụng giải trí - Chương III: Ảnh - Lê Tấn Hùng
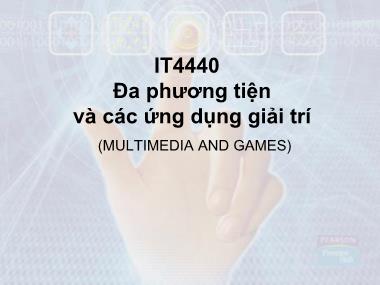
(MULTIMEDIA AND GAMES) IT4440 Đa phương tiện và các ứng dụng giải trí Nội dung môn học Tuần Chủ đề Số tiết 1 Giới thiệu về môn học 1 – 5 Phần I. Tổng quan về thông tin đa phương tiện và các kỹ thuật xử lý 15 1 Chương I: Nhập môn Multimedia 1 1 Chương II: Một số kiến thức cơ bản 1 2 Chương III: Ảnh 4 3 Chương IV: Màu 3 4 Chương V: Video 3 5 Chương VI: Audio 3 6 – Phần II. Một số ứng dụng đa phương tiện Chương V: Multimedia- ứng dụng và giải trí Chương VI: Ứng dụng web Chương VII: Ứng dụng mobile Chương VIII: Ứng dụng 3D Chương IX: Ứng dụng Game Bảo vệ Bài tập lớn, Tổng kết ôn tập Nội dung môn học Tuần Chủ đề Số tiết 1 Giới thiệu về môn học 1 – 5 Phần I. Tổng quan về thông tin đa phương tiện và các kỹ thuật xử lý 15 1 Chương I: Nhập môn Multimedia 1 1 Chương II: Một số kiến thức cơ bản 1 2 Chương III: Ảnh 4 3 Chương IV: Màu 3 4 Chương V: Video 3 5 Chương VI: Audio 3 6 – Phần II. Một số ứng dụng đa phương tiện Chương V: Multimedia- ứng dụng và giải trí Chương VI: Ứng dụng web Chương VII: Ứng dụng mobile Chương VIII: Ứng dụng 3D Chương IX: Ứng dụng Game Bảo vệ Bài tập lớn, Tổng kết ôn tập Mục tiêu của chương Quá trình tạo ảnh Biểu diễn và lưu trữ ảnh Nén ảnh Một số kỹ thuật xử lý ảnh cơ bản Một số công cụ xử lý ảnh Tổng kết chương Tài liệu tham khảo Chương III: Ảnh Người học sẽ: Được trang bị kiến thức về quá trình tạo ảnh, biểu diễn, nén và lưu trữ ảnh Được giới thiệu một số kỹ thuật xử lý ảnh cơ bản, một số công cụ xử lý Sau khi kết thúc chương, người học : Nắm được kiến thức cơ bản của tạo ảnh, biểu diễn, lưu trữ ảnh Biết vận dụng một số kỹ thuật, công cụ xử lý ảnh để thực hành xử lý một số ảnh cụ thể III.1 Mục tiêu của chương III.2 Quá trình tạo ảnh Ảnh (Bimmaped Image) được tạo ra như thế nào ? III.2 Quá trình tạo ảnh III.2 Quá trình tạo ảnh Ống kính và điểm nhìn xác định phối cảnh Độ mở ống kính và tốc độ đóng quyết định độ sáng ảnh Độ mở và các hiệu ứng khác quyết định độ sâu ảnh Film hay cảm biến cho phép lưu ảnh III.2 Quá trình tạo ảnh Bộ cảm biến, film sẽ « cảm» ánh sáng từ mọi phía III.2 Quá trình tạo ảnh Pinhole Camera model: ánh sáng đi qua một lỗ nhỏ World Camera Digitizer Digital image Source : Tal Hassner. Computer Vision. Weizmann Institute of Science (Israel). III.2 Quá trình tạo ảnh Quá trình tạo ảnh số (digital image) III.2 Quá trình tạo ảnh CCD: Charge Coupled Device (Thiết bị tích điện kép) Tiếp nhận ánh sáng tới Ánh sáng tới được chuyển thành các tín hiệu điện Năng lượng của tín hiệu điện tỷ lệ thuận với lượng ánh sáng tới Có các bộ lọc để tăng tính chọn lựa III.2 Quá trình tạo ảnh KAF-1600 - Kodak. Cảm biến quang CCD III.2 Quá trình tạo ảnh Tạo ảnh màu như thế nào ? III.2 Quá trình tạo ảnh Minh họa quá trình tạo ảnh RGB Mỗi điểm ảnh trên cảm biến được coi như một thùng chứa Các photon ánh sáng sẽ rơi vào các thùng chứa. Cường độ sáng tỷ lệ thuận với số photon ánh sang có trong thùng chứa III.2 Quá trình tạo ảnh Tại sao lại có hai Green, một Blue và một Red trong mô hình Bayer ? Cảm biến Bayer và Foveon III.2 Quá trình tạo ảnh Thực sự thì camera đã « nhìn » thấy gì ? III.2 Quá trình tạo ảnh III.2 Quá trình tạo ảnh Để tạo thành bức ảnh giống như ta nhìn thấy, cần phải thực hiện bước « Demosaicing » Đối với mô hình Bayer, kết hợp 4 phần tử liền kề để tạo thành một điểm ảnh có giá trị RGB III.3 Ảnh số: Biểu diễn Các giá trị điện thế mà ta thu được tương ứng với đáp ứng của bộ cảm biến quang đối với môi trường quan sát Các giá trị này (Voltage) là các giá trị liên tục (Analog) Các giá trị này sẽ được số hóa để cho ta mảng các điểm, mỗi điểm có 3 giá trị (R, G, B) => Ảnh số Light → Electric charge → Number III.3 Ảnh số: Biểu diễn ảnh số được tạo ra như thế nào ? III.3 Ảnh số: Biểu diễn Digitization = Sampling + Quantization 23 Source : Gonzalez and Woods. Digital Image Processing. Prentice-Hall, 2002. III.3 Ảnh số: Biểu diễn Lấy mẫu và lượng tử hóa Cường độ sáng của đường quét ngang Ảnh gốc Lấy mẫu Lượng tử hóa Lấy mẫu ảnh bị giới hạn bởi kích thước của cảm biến (kích thước của ma trận điểm ảnh trên cảm biến) Lượng tử hóa bị hạn chế bởi số mức ánh sáng định nghĩa trong một giải nào đó Source : Gonzalez and Woods. Digital Image Processing. Prentice-Hall, 2002. III.3 Ảnh số: Biểu diễn Source : Gonzalez and Woods. Digital Image Processing. Prentice-Hall, 2002. Ảnh tương tự trên cảm biến Ảnh sau khi lấy mẫu và lượng tử hóa III.3 Ảnh số: Biểu diễn Ảnh được biểu diễn bởi một ma trận kích thước MxN, tương ứng với số điểm ảnh của bộ cảm biến quang Mỗi phần tử của ảnh sẽ có 1 đến 3 giá trị tùy thuộc vào ảnh mức xám (đen trắng) hay ảnh màu Các giá trị là một số nguyên nằm trong khoảng [Lmin, Lmax] Tổng số bít cần thiết để biểu diễn các mức xám trong khoảng L là K sao cho: L= 2K Tổng số bit cần để lưu trữ một ảnh là: MxNxK (bít) III.3 Ảnh số: Biểu diễn III.3 Ảnh số: Độ phân giải của ảnh Độ phân giải ảnh là gì ? Độ phân giải trong không gian Là phần tử nhỏ nhất nhìn thấy được (kích thước điểm ảnh) Độ phân giải theo mức xám Sự thay đổi màu sắc nhỏ nhất có thể quan sát đươc Một ảnh có độ phân giải không gian M X N điểm ảnh có độ phân giải mức xám là K bits hay L mức xám III.3 Ảnh số: Độ phân giải của ảnh III.3 Ảnh số: Độ phân giải của ảnh Độ phân giải không gian Độ phân giải mức xám III.3 Ảnh số: Độ phân giải của ảnh Kích thước vật lý của một ảnh khi nó được hiển thị phụ thuộc vào mật độ điểm ảnh trên thiết bị hiển thị (dpi = dots per inch) III.3 Ảnh số: Độ phân giải của ảnh III.3 Ảnh số: Độ phân giải của ảnh Hầu hết các định dạng file ảnh để lưu độ phân giải ảnh cùng với giá trị các điểm ảnh, thường là độ phân giải của thiết bị thu nhận (camera) III.3 Ảnh số: Độ phân giải của ảnh III.3 Ảnh số: Lưu trữ 64 60 69 100 149 151 176 182 179 65 62 68 97 145 148 175 183 181 65 66 70 95 142 146 176 185 184 66 66 68 90 135 140 172 184 184 66 64 64 84 129 134 168 181 182 59 63 62 88 130 128 166 185 180 60 62 60 85 127 125 163 183 178 62 62 58 81 122 120 160 181 176 63 64 58 78 118 117 159 180 176 Mức xám - 8 bits: 0 - đen 255 - trắng 210 209 204 202 197 247 143 71 64 80 84 54 54 57 58 206 196 203 197 195 210 207 56 63 58 53 53 61 62 51 201 207 192 201 198 213 156 69 65 57 55 52 53 60 50 216 206 211 193 202 207 208 57 69 60 55 77 49 62 61 221 206 211 194 196 197 220 56 63 60 55 46 97 58 106 209 214 224 199 194 193 204 173 64 60 59 51 62 56 48 204 212 213 208 191 190 191 214 60 62 66 76 51 49 55 214 215 215 207 208 180 172 188 69 72 55 49 56 52 56 209 205 214 205 204 196 187 196 86 62 66 87 57 60 48 208 209 205 203 202 186 174 185 149 71 63 55 55 45 56 207 210 211 199 217 194 183 177 209 90 62 64 52 93 52 208 205 209 209 197 194 183 187 187 239 58 68 61 51 56 204 206 203 209 195 203 188 185 183 221 75 61 58 60 60 200 203 199 236 188 197 183 190 183 196 122 63 58 64 66 205 210 202 203 199 197 196 181 173 186 105 62 57 64 63 x = 58 59 60 61 62 63 64 65 66 67 68 69 70 71 72 41 42 43 44 45 46 47 48 49 50 51 52 53 54 55 y = III.3 Ảnh số: Lưu trữ III.3 Ảnh số: Biểu diễn và lưu trữ Ảnh là một tín hiệu 2D (x, y) Về mặt toán học: Ảnh là một ma trận biểu diễn tín hiệu Đối với người dùng: Ảnh chứa các thông tin ngữ nghĩa (khung cảnh đường phố) Phân loại ảnh Ảnh tự nhiên – thu nhận từ các thiết bị camera, microscope, tomography, infrared, satellite, Ảnh nhân tạo – Đồ họa máy tính (computer graphics), thực tại ảo (virtual reality) Ảnh tự nhiên ảnh nhân tạo Ảnh nhân tạo Ảnh mức xám I(x,y) in [0..255] Ảnh nhị phân I(x,y) in {0 , 1} Ảnh màu IR(x,y) IG(x,y) IB(x,y) Source : Tal Hassner. Computer Vision. Weizmann Institute of Science (Israel). Phân loại ảnh Ảnh màu trong hệ tọa độ RGB Bên cạnh hệ tọa độ màu RGB ta còn có các hệ tọa độ màu khác Source : Tal Hassner. Computer Vision. Weizmann Institute of Science (Israel). III.4 Nén ảnh (image compression) Tại sao cần phải nén ? Tại sao ta có thể nén ảnh ? Các phương pháp thường dùng để nén ảnh ? Tại sao cần phải nén ? Lượng dữ liệu ngày càng lớn Các yêu cầu về lưu trữ và truyền thông DVD Video conference Printer Tốc độ truyền dữ liệu cinema không nén:1Gbps III.4 Nén ảnh (image compression) Tại sao ta có thể nén ảnh ? Sự dư thừa thông tin theo không gian, thời gian, tần số Các pixel lân cận không độc lập nhưng tương quan lẫn nhau III.4 Nén ảnh (image compression) III.4 Nén ảnh (image compression) Dư thừa thông tin trong không gian (Spatial Redundancy) III.4 Nén ảnh (image compression) Dư thừa thông tin theo cường độ sáng I2 I1 Theo định luật Weber: sai khác DI = I1 – I2, chỉ có thể phân biệt được khi DI/I1 đủ lớn The high (bright) values need a less accurate representation compared to the low (dark) values Weber’s law holds for all human senses! III.4 Nén ảnh (image compression) Dư thừa thông tin theo tần số Hệ thống thị giác của con người cũng giống như một bộ lọc: Các thành phần tần số quá cao sẽ bị bỏ qua Nguyên lý nén ảnh là gì ? Chỉ dữ lại thông tin III.4 Nén ảnh (image compression) REDUNDANTDATA INFORMATION DATA = INFORMATION + REDUNDANT DATA Nguyên lý nén ảnh là gì ? Chỉ dữ lại thông tin Vậy làm thế nào để phát hiện ra sự dư thừa thông tin phục vụ trong các giải thuật nén ảnh III.4 Nén ảnh (image compression) Mô hình chung của nén ảnh trong các hệ thống truyền và lưu trữ dữ liệu III.4 Nén ảnh (image compression) Coder: (en)coder + decoder = codec Source encoder: removes redundancy Channel encoder: adds redundancy A/D, D/A, en/decryption optional Only deal with the source coder Bộ mã hóa nguồn tin Coded bit-string Coded bit-string Transformation Input Info. Reconstructed Information Codeword decoder Inverse transformation Codeword assignment Quantization Transformation: new representation of data Differential coding, transform coding (MM2) Quantization: In-reversible process => lossy coding Codeword assignment (entropy coding): Info. Theory: Huffman, run length, arithmetic, dictionary coding After transformation and quantization => source symbols: s1, s2, s3,, sn The symbols need to be represented by bits Remove the redundancy in the symbols (lossless) Methods: Run length, Huffman, arithmetic, modifications, dictionary (LZW: zip, gif, tiff, pdf,..) Quick introduction to run length and Huffman coding Codeword assignment Input: 7,7,7,7,7,13,90,9,9,9,2,1,1,0,5,= 15 Byte RLE: 5,7,13,90,3,9,2,2,1,0,5,= 11 Byte How to distinguish between values and counts? One value of a byte to indicate a count, e.g. 0 or 255, e.g. 255: 255,5,7,13,90,255,3,9,2,255,2,1,0,5,= 14 Byte One bit to indicate count [1] and value [0] for 8 values => [10001001],5,7,13,90,3,9,2,2,[000]1,0,5.. ~ 12,5 Byte Run length coding Arrange symbols: p(s2) > p(s5) > > p(s3) li = length in bits of the i’th symbol si Key idea: use fewer bits to code the most likely symbols: l2 < l5 < < l3 Huffman coding Huffman coding Algorithm: Arrange symbols Loop: Combine the two symbols with lowest probabilities into a new symbol Assign one bit and update probabilities Re-arrange symbols Codewords: back trace S1 ( 0.30 ) S6 ( 0.25 ) S3 ( 0.20 ) S2 ( 0.10 ) S5 ( 0.10 ) S4 ( 0.05 ) S1 ( 0.30 ) S6 ( 0.25 ) S3 ( 0.20 ) S5,4 ( 0.15 ) S2 ( 0.10 ) S1 ( 0.30 ) S6 ( 0.25 ) S5,4,2 ( 0.25 ) S3 ( 0.20 ) S5,4,2,3 ( 0.45 ) S1 ( 0.30 ) S6 ( 0.25 ) S1,6 ( 0.55 ) S5,4,2,3 ( 0.45 ) S ( 1.0 ) 0 0 0 0 0 1 1 1 1 1 "Joint Photographic Expert Group". Voted as international standard in 1992. Works with color and grayscale images, e.g., satellite, medical, ... Lossy and lossless III.4 Nén ảnh: JPEG 1987: ITU + ISO => international standard for still image compression, due to grows in the PC market: JPEG = Joint Photographic Expert Group Goal: non-binary images keeping a good to excellent image quality First standard in 1992 JPEG is NOT an algorithm but rather a framework with several algorithms and user-settings III.4 Nén ảnh: JPEG First generation JPEG uses DCT + Run length Huffman entropy coding. Second generation JPEG (JPEG2000) uses wavelet transform + Bit plane coding + Arithmetic entropy coding. III.4 Nén ảnh : JPEG Các thông tin tần số cao có thể bị loại bỏ mà không làm mất mát thông tin quan sát vì mắt người không cảm nhận được những hiệu ứng do các thành phần tần số cao mang lại một cách chính xác Ảnh được chuyển sang miền tần số sử dụng phép biến đổi Cosin rời rạc - Discrete Cosine Transform (DCT). Phép biến đổi DCT thường được áp dụng cho các khối pixel kích thước 8 × 8. Việc áp dụng DCT không làm giảm kích thước của dữ liệu, vì số các hệ số của DCT cũng bằng tổng số pixel của khối (64). Tuy nhiên, các hệ số của DCT được lượng tử hóa, vì thế số bit cần thiết để biểu diễn các hệ số DCT sẽ giảm đi. Việc lượng tử hỏa sẽ làm biến mất một số thông tin. III.4 Nén ảnh: JPEG DCT is similar to DFT, but can provide a better approximation with fewer coefficients The coefficients of DCT are real valued instead of complex valued in DFT. III.4 Nén ảnh: JPEG Tại sao là DCT mà không phải là DFT ? III.4 Nén ảnh: JPEG • Each 8x8 block can be looked at as a weighted sum of these basis functions. • The process of 2D DCT is also the process of finding those weights. The 64 (8 X 8) DCT Basis Functions Zig-zag Scan DCT Blocks Why? -- To group low frequency coefficients in top of vector. Maps 8 x 8 to a 1 x 64 vector. Ảnh gốc Ảnh JPEG 27:1 JPEG2000 27:1 Original image 512 x 512 x 8 bits = 2,097,152 bits JPEG 27:1 reduction =77,673 bits Ví dụ về nén JPEG Bài tập Why is it possible to compress images? Explain the JPEG framework What is the compression factor of this luminance DCT- block? 130 5 4 -34 11 -17 14 10 2 47 6 1 -8 14 21 22 -19 1 -2 -3 6 3 -1 -21 -3 5 -1 5 -1 -2 17 11 7 -9 2 10 9 1 -4 9 2 4 -6 -11 12 -7 40 -17 -1 -12 -3 1 9 14 57 34 22 5 4 -2 33 -21 14 -27 GIF PNG JPEG TiFF BMP Các định dạng file ảnh là một định dạng tập tin hình ảnh bitmap cho các hình ảnh dùng ít hơn 256 màu sắc khác nhau và các hoạt hình dùng ít hơn 256 màu cho mỗi khung hình. GIF là định dạng nén dữ liệu đặc biệt hữu ích cho việc truyền hình ảnh qua đường truyền lưu lượng nhỏ. Định dạng này được CompuServe cho ra đời vào năm 1987 và nhanh chóng được dùng rộng rãi trên World Wide Web cho đến nay. Graphics Interchange Format - GIF Là một dạng hình ảnh sử dụng phương pháp nén dữ liệu mới - không làm mất đi dữ liệu gốc. PNG được tạ ... BMP BẢNG TỔNG KẾT CÁC ĐỊNH DẠNG FILE Color data mode - Bits per pixel TIF RGB - 24 or 48 bits, Grayscale - 8 or 16 bits, Indexed color - 1 to 8 bits, Line Art (bilevel)- 1 bitFor TIF files, most programs allow either no compression or LZW compression (lossless, but is less effective for 24 bit color images). Adobe Photoshop also provides JPG or ZIP compression too (but which greatly reduces third party compatibility of TIF files). "Document programs" allow ITCC G3 or G4 compression for 1 bit text (Fax is G3 or G4 TIF files), which is lossless and tremendously effective (small). PNG RGB - 24 or 48 bits, Grayscale - 8 or 16 bits, Indexed color - 1 to 8 bits, Line Art (bilevel) - 1 bitPNG uses ZIP compression which is lossless, and slightly more effective than LZW (slightly smaller files). PNG is a newer format, designed to be both verstile and royalty free, back when the LZW patent was disputed. JPG RGB - 24 bits, Grayscale - 8 bitsJPEG always uses lossy JPG compression, but its degree is selectable, for higher quality and larger files, or lower quality and smaller files. GIF Indexed color - 1 to 8 bitsGIF uses lossless LZW compression, effective on indexed color. GIF files contain no dpi information for printing purposes. File format and purpose Photographic Images Graphics, including Logos or Line art Properties Photos are continuous tones, 24 bit color or 8 bit Gray, no text, few lines and edges Graphics are often solid colors, up to 256 colors, with text or lines and sharp edges For Unquestionable Best Quality TIF or PNG (lossless compression and no JPG artifacts) PNG or TIF (lossless compression, and no JPG artifacts) Smallest File Size JPG with a higher Quality factor can be decent. TIF LZW or GIF or PNG (graphics/logos without gradients normally permit indexed color of 2 to 16 colors for smallest file size) Maximum Compatibility (PC, Mac, Unix) TIF or JPG TIF or GIF Worst Choice 256 color GIF is very limited color, and is a larger file than 24 bit JPG JPG compression adds artifacts, smears text and lines and edges III.5 Một số kỹ thuật xử lý ảnh cơ bản Thế nào là xử lý ảnh ? Xử lý Ảnh đầu ra Ảnh đầu vào Các phép xử lý cơ bản Các bộ lọc tuyến tính Blurring Sharpening Edge detection Wiener denoising Các bộ lọc phi tuyến Median filter Bilateral filter Cross-bilateral filter III.5 Một số kỹ thuật xử lý ảnh cơ bản Lược đồ ảnh Lược đồ ảnh là phân bố các giá trị mức xám (màu ) của một ảnh H(k) = tổng số pixel trong ảnh có giá trị k Number of pixels Gray level PI(k) k PI(k) k 1 1 0.5 PI(k) k 0.1 Image dynamic range = [min_value, max_value] Source : Tal Hassner. Computer Vision. Weizmann Institute of Science (Israel). Lược đồ ảnh Luminance của một ảnh được định nghĩa là giá trị trung bình của tất cả các mức xám trong ảnh Trong ảnh dưới đây, chỉ có luminance thay đổi Source : Eric Favier. L'analyse et le traitement des images. ENISE. Luminance (độ sáng) The contrast can be defined in many different ways : Standard deviation of the gray levels Variation between the min and max gray level Contrast (độ tương phản) Hai ảnh dưới đây khác nhau về độ tương phản Contrast (độ tương phản) Source : Gonzalez and Woods. Digital Image Processing. Prentice-Hall, 2002. Ví dụ về độ tương phản của ảnh Có nhiều phương pháp Chuyển đổi tuyến tính Piecewise linear transform Non-linear transform Histogram equalization Source : Caroline Rougier. Traitement d'images (IFT2730). Univ. de Montréal. Tăng cường độ tương phản ảnh I’ I min max 255 0 I(i,j) I’(i,j) 1 , 0 min max min) ) , ( ( min) ) , ( ( min max 255 ) , ( ' j i I with j i I i i I Source : Caroline Rougier. Traitement d'images (IFT2730). Univ. de Montréal. Biến đổi tuyến tính 255 255 min max 255 255 % 0 255 min max 0 255 Source : Caroline Rougier. Traitement d'images (IFT2730). Univ. de Montréal. Biến đổi tuyến tính 0 50 100 150 200 250 0 500 1000 1500 2000 2500 3000 3500 0 50 100 150 200 250 0 500 1000 1500 2000 2500 3000 3500 Source image More contrasted image Source : Tal Hassner. Computer Vision. Weizmann Institute of Science (Israel). Cân bằng lược đồ xám Histogram equalization can improve the image contrast where histogram dynamic correction is of no use Cân bằng lược đồ xám If we take the same image with different contrasts, histogram equalization will give the same results for all images Source : Gonzalez and Woods. Digital Image Processing. Prentice-Hall, 2002. Cân bằng lược đồ xám Logical operators can be applied to images AND OR = = Source : Gonzalez and Woods. Digital Image Processing. Prentice-Hall, 2002. Một số toán tử logic (AND, OR) If f and g are two images, the pixelwise addition R is defined as: R(x,y) = Min( f(x,y)+g(x,y) ; 255 ) Image addition is used to lower the noise in a serie of images increase the luminance by adding the image to itself Source : Eric Favier. L'analyse et le traitement des images. ENISE. Cộng ảnh The pixelwise substraction of two images f and g is: S(x,y) = Max( f(x,y)- g(x,y) ; 0 ) Image substraction is used to detect defaults detect motion in images Source : Eric Favier. L'analyse et le traitement des images. ENISE. Trừ ảnh The multiplication S of an image f by a ratio (factor) is defined as: S(x,y) = Max( f(x,y)*ratio ; 255) Image multiplication can be used to increase the contrast or the luminosity Source : Eric Favier. L'analyse et le traitement des images. ENISE. Nhân ảnh x1,5 = x1,2 = 93 0.5*F(x,y) + 0.5*G(x,y) F(x,y) G(x,y) F(x,y) - G(x,y) G(x,y) - F(x,y) Source : www.nte.montaigne.u-bordeaux.fr/SuppCours/5314/Dai/TraitImage01-02.ppt Một số phép toán trên các ảnh Các phép xử lý cơ bản Các bộ lọc tuyến tính Blurring Sharpening Edge detection Các bộ lọc phi tuyến Median filter Bilateral filter Cross-bilateral filter III.5 Một số kỹ thuật xử lý ảnh cơ bản Các bộ lọc làm mịn ảnh (Blurring) In general, for symmetry f(u,v) = f(u) f(v) You might want to have some fun with asymmetric filters We will use a Gaussian blur Blur width sigma depends on kernel size n (3,5,7,11,13,19) Spatial Frequency 2 2 1 ( ) exp ( / 2) / 2 22 u f u floor n Slide credit: Ravi Ramamoorthi Discrete Filtering, Normalization Gaussian is infinite In practice, finite filter of size n (much less energy beyond 2 sigma or 3 sigma). Must renormalize so entries add up to 1 Simple practical approach Take smallest values as 1 to scale others, round to integers Normalize. E.g. for n = 3, sigma = ½ 2 2 2 2 2 2 1 2 ( , ) exp exp 2 2 2 u v f u v u v 0.012 0.09 0.012 1 7 1 1 0.09 0.64 0.09 7 54 7 86 0.012 0.09 0.012 1 7 1 Slide credit: Ravi Ramamoorthi Slide credit: Ravi Ramamoorthi Slide credit: Ravi Ramamoorthi Sharpening (làm sắc nét) original 0 2.0 0 0.33 Sharpened original Slide credit: Bill Freeman Sharpening example co ef fi ci en t -0.3 original 8 Sharpened (differences are accentuated; constant areas are left untouched). 11.2 1.7 -0.25 8 Slide credit: Bill Freeman Sharpening Filter Unlike blur, want to accentuate high frequencies Take differences with nearby pixels (rather than avg) 1 2 1 1 ( , ) 2 19 2 7 1 2 1 f x y Sharpening before after Slide credit: Bill Freeman Edge Detection Edge Detection Edge Detection Complicated topic: subject of many PhD theses Here, we present one approach (Sobel edge detector) Step 1: Convolution with gradient (Sobel) filter Edges occur where image gradients are large Separately for horizontal and vertical directions Step 2: Magnitude of gradient Norm of horizontal and vertical gradients Step 3: Thresholding Threshold to detect edges Slide credit: Ravi Ramamoorthi Các phép xử lý cơ bản Các bộ lọc tuyến tính Blurring Sharpening Edge detection Các bộ lọc phi tuyến Median filter Bilateral filter Cross-bilateral filter III.5 Một số kỹ thuật xử lý ảnh cơ bản Median filter Replace each pixel by the median over N pixels (5 pixels, for these examples). Generalizes to “rank order” filters. 5-pixel neighborhood In: Out: In: Out: Spike noise is removed Monotonic edges remain unchanged Median([1 7 1 5 1]) = 1 Mean([1 7 1 5 1]) = 2.8 Median filtering results Best for salt and pepper noise Panorama Image Blending Image Warping Image Morphing Một số thao tác với ảnh Panorama Image Blending Image Warping Image Morphing Một số thao tác với ảnh Introduction Are you getting the whole picture? Compact Camera Field of View (FOV) = 50 x 35° Introduction Are you getting the whole picture? Compact Camera FOV = 50 x 35° Human FOV = 200 x 135° Introduction Are you getting the whole picture? Compact Camera FOV = 50 x 35° Human FOV = 200 x 135° Panoramic Mosaic = 360 x 180° Why “Recognising Panoramas”? 1D Rotations (q) Ordering matching images • 2D Rotations (q, f) – Ordering matching images Why “Recognising Panoramas”? 1D Rotations (q) Ordering matching images • 2D Rotations (q, f) – Ordering matching images Mosaics: stitching images together virtual wide-angle camera Slide credit: F. Durand How to do it? Basic Procedure Take a sequence of images from the same position Rotate the camera about its optical center Compute transformation between second image and first Transform the second image to overlap with the first Blend the two together to create a mosaic If there are more images, repeat but wait, why should this work at all? What about the 3D geometry of the scene? Why aren’t we using it? Slide credit: F. Durand Panorama Image Blending Image Warping Image Morphing Một số thao tác với ảnh Best blending examples Paul Gentry Best blending examples Marco Best blending examples Roger Xue Best blending examples Alfredo Best blending examples Merve Best blending examples Alex Rubinsteyn Một số thao tác với ảnh Blending image được thực hiện như thế nào Sinh viên về nhà tự tìm hiểu Panorama Image Blending Image Warping Image Morphing Một số thao tác với ảnh Morphing = Object Averaging The aim is to find “an average” between two objects Not an average of two images of objects but an image of the average object! How can we make a smooth transition in time? Do a “weighted average” over time t How do we know what the average object looks like? We haven’t a clue! But we can often fake something reasonable Usually required user/artist input Slide credit: Alyosha Efros Idea #1: Cross-Dissolve Interpolate whole images: Imagehalfway = (1-t)*Image1 + t*image2 This is called cross-dissolve in film industry But what is the images are not aligned? Slide credit: Alyosha Efros Idea #2: Align, then cross-disolve Align first, then cross-dissolve Alignment using global warp – picture still valid Slide credit: Alyosha Efros Panorama Image Blending Image Warping Image Morphing Một số thao tác với ảnh Dog Averaging What to do? Cross-dissolve doesn’t work Global alignment doesn’t work Cannot be done with a global transformation (e.g. affine) Any ideas? Feature matching! Nose to nose, tail to tail, etc. This is a local (non-parametric) warp Slide credit: Alyosha Efros Idea #3: Local warp, then cross-dissolve Morphing procedure: for every t, 1. Find the average shape (the “mean dog”) local warping 2. Find the average color Cross-dissolve the warped images Slide credit: Alyosha Efros Local (non-parametric) Image Warping Need to specify a more detailed warp function Global warps were functions of a few (2,4,8) parameters Non-parametric warps u(x,y) and v(x,y) can be defined independently for every single location x,y! Once we know vector field u,v we can easily warp each pixel (use backward warping with interpolation) Slide credit: Alyosha Efros Image Warping – non-parametric Move control points to specify a spline warp Spline produces a smooth vector field Slide credit: Alyosha Efros Warp specification - dense How can we specify the warp? Specify corresponding spline control points • interpolate to a complete warping function But we want to specify only a few points, not a grid Slide credit: Alyosha Efros Warp specification - sparse How can we specify the warp? Specify corresponding points • interpolate to a complete warping function • How do we do it? How do we go from feature points to pixels? Slide credit: Alyosha Efros Triangular Mesh 1. Input correspondences at key feature points 2. Define a triangular mesh over the points Same mesh in both images! Now we have triangle-to-triangle correspondences 3. Warp each triangle separately from source to destination How do we warp a triangle? 3 points = affine warp! Just like texture mapping Slide credit: Alyosha Efros III.6 Một số công cụ thao tác ảnh Em đã từng sử dụng một công cụ nào để thao tác ảnh chưa ? Công cụ gì ? III.6 Một số công cụ thao tác ảnh Photoshop is the de facto industry standard; The Gimp is an Open Source alternative. Image Magick can be used for command-line processing. Others ? Bitmapped images are manipulated to correct technical deficiencies, alter the content or create artificial compositions Images are often organized into layers, which are like overlaid sheets that may have transparent areas. Layers are used for compositing or experimenting with different versions of an image. Một số thao tác với công cụ Compositing layers Areas may be selected by drawing with marquee and lasso tools or a Bézier pen, or selected on the basis of colour similarity or edges using a magic wand or magnetic lasso. Magic wand selection Magic lasso selection Any selection defines a mask – the area that is not selected. Masked areas of the image are protected from changes. A greyscale mask, which is partially transparent, is an alpha channel. An alpha channel can be associated with a layer as a layer mask, and used for effects such as knock-outs and vignettes Compositing with a layer mask Vignetting an image In pixel point processing, each pixel’s new value depends only on its old value. Brightness, contrast and levels are relatively crude pixel point adjustments. Tăng cường độ tương phản và độ sáng Tổng kết chương Bài giảng Alain Boucher (Image Processing and Computer Vision – Chapter 2) Bài giảng Rob Fergus (Computational Photography – Chapter 1, 2) Ebook, Digital Multimedia – Chapter 4 Bài giảng Trần Thị Thanh Hải, CSDL đa phương tiện, Image, 2010 Tài liệu tham khảo của chương
File đính kèm:
bai_giang_da_phuong_tien_va_cac_ung_dung_giai_tri_chuong_iii.pdf